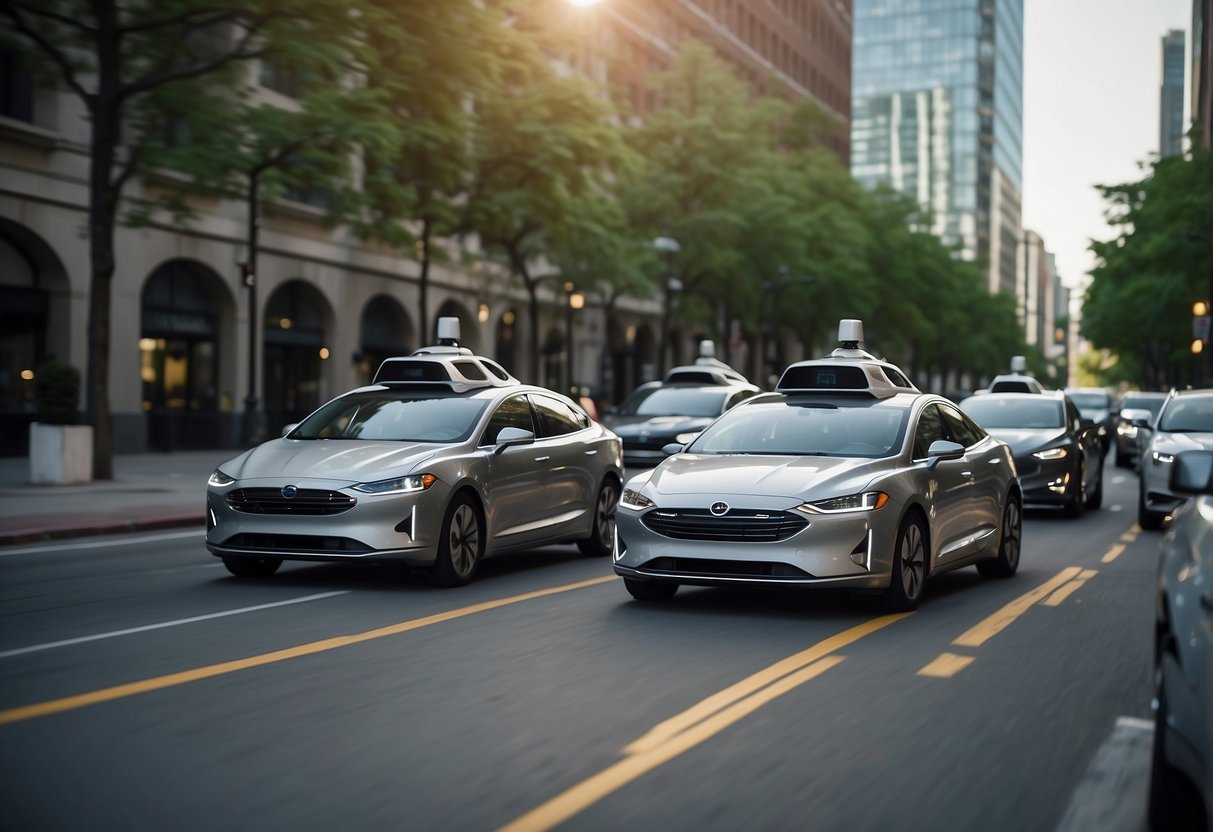
Connectivity and V2X Communication
Connectivity and V2X Communication play crucial roles in enhancing the safety and efficiency of self-driving cars. These systems enable vehicles to interact with their surroundings and other vehicles, facilitating better decision-making and advanced driving capabilities.
Vehicle to Infrastructure
Vehicle to Infrastructure (V2I) communication allows autonomous cars to exchange information with traffic lights, road signs, and other road infrastructure. This connectivity helps optimize traffic flow and reduce congestion.
For instance, a self-driving car can receive real-time updates on traffic signal timings, allowing it to adjust speed and avoid unnecessary stops. Infrastructure can also transmit information about road conditions, upcoming construction zones, or accidents, enabling the vehicle to make informed route adjustments. V2I systems thus significantly contribute to safer and more efficient driving experiences.
Vehicle to Vehicle
Vehicle to Vehicle (V2V) communication enables autonomous cars to share data such as speed, position, and direction with other nearby vehicles. This instantaneous exchange of information enhances collision avoidance and overall traffic coordination.
For example, if a car ahead suddenly brakes, V2V communication quickly alerts following vehicles, reducing the risk of rear-end collisions. It can also support synchronized maneuvers like lane changes and merging, improving traffic flow. V2V technology is fundamental in creating a connected and cooperative driving environment, essential for autonomous vehicle operations.
Real-World Testing and Deployment
Autonomous vehicles are advancing rapidly through pilot programs in various cities and regions. Analyzing data from these programs provides crucial insights into performance, safety, and public reception.
Pilot Programs and Case Studies
Numerous pilot programs have been initiated to test self-driving cars in different environments. Companies like Waymo and Tesla have operated extensive test programs in cities ranging from Phoenix to San Francisco. These pilots help to identify how autonomous vehicles handle real-world conditions such as traffic variability, weather changes, and pedestrian behavior.
Case studies from these programs highlight both achievements and challenges. For instance, Waymo has achieved significant mileage with minimal accidents, showcasing the reliability of their technology. Meanwhile, some incidents reveal areas needing improvement, such as better handling of complex urban settings.
Collaborations with local governments and transit authorities also contribute to these studies. By working together, regulators and companies establish frameworks for safe deployment, addressing legal and ethical considerations. These interactions provide a comprehensive understanding of how autonomous vehicles integrate into existing transportation systems.
Analyzing Field Data
Analyzing data collected from real-world testing is critical for refining autonomous technology. Data points include vehicle response times, navigation accuracy, and safety metrics. This analysis helps engineers improve algorithms that control everything from obstacle avoidance to emergency maneuvers.
Safety remains a primary focus in data analysis. Identifying the root causes of any incidents ensures that future deployments are safer. For example, data showing how vehicles perform in adverse weather conditions can lead to better sensor and software enhancements.
Data analysis also informs regulatory decisions. Governments use this information to create guidelines and policies that ensure public safety while fostering innovation. Effective data interpretation allows for iterative improvements, making each new deployment phase safer and more efficient than the last.