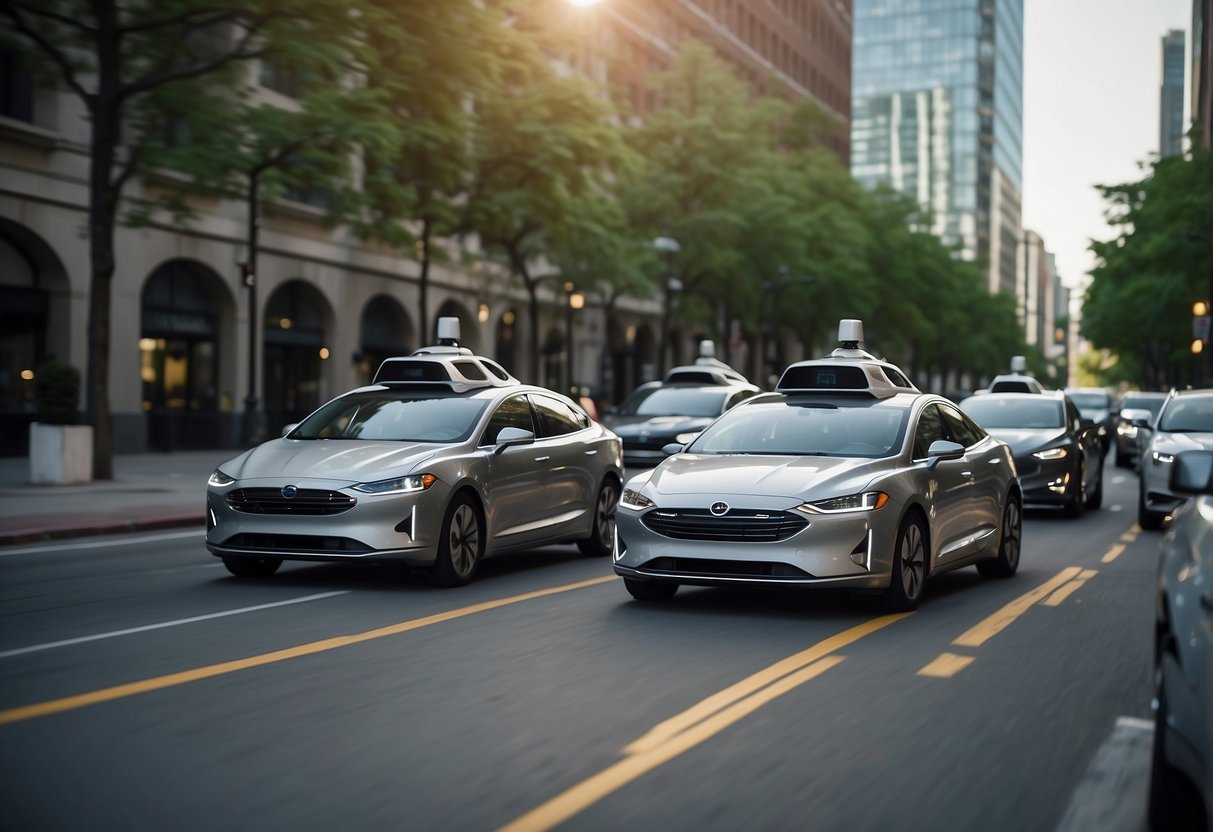
Mapping and Localization Techniques
Mapping and localization involve creating and updating detailed maps and determining the precise position of the vehicle within these maps. High-definition maps (HD maps) are essential, containing comprehensive details about road geometry, lane markings, traffic signs, and other fixed elements. These maps are continuously updated to reflect real-time changes. For localization, self-driving cars use a combination of GPS, IMU (Inertial Measurement Unit), and sensor data to pinpoint their exact location. This precise localization is crucial for safe navigation, lane keeping, and adapting to changes in the environment.
Path Planning and Decision Making
Path planning and decision-making systems enable self-driving cars to determine the optimal route and make real-time decisions. The path planning process involves calculating the safest and most efficient trajectory from the current position to the destination. This requires considering various factors like traffic conditions, road obstacles, and legal constraints. Decision-making algorithms analyze data from sensors and maps to make split-second decisions, such as changing lanes, stopping at signals, or avoiding obstacles. These systems use advanced machine learning techniques to continuously improve and adapt to different driving scenarios, ensuring the vehicle operates safely and efficiently in diverse environments.
Hardware and Software Integration
Self-driving cars rely on a blend of advanced hardware and software to navigate safely and effectively. Key elements include sophisticated computer systems and robust safety features.
Onboard Computer Systems
The heart of an autonomous vehicle is its onboard computer system. These systems process vast amounts of data from sensors, cameras, and other inputs. High-performance processors and GPUs handle real-time decision-making, route planning, and obstacle detection.
These computers use machine learning algorithms to interpret sensor data, adjust driving tactics, and enhance performance. Cloud connectivity supports software updates, ensuring the latest improvements are always in place. Efficient data processing and communication between components are paramount.
Safety Features and Redundancies
Safety is critical in autonomous vehicles, achieved through multiple redundancies in both hardware and software. Sensors such as LiDAR, radar, and cameras work in unison to provide overlapping fields of view.
Redundant systems ensure the vehicle can still function in case one component fails. Autonomous cars also incorporate emergency protocols, including safe stopping mechanisms and alert systems. Regular software updates address vulnerabilities and improve safety algorithms, helping to maintain and enhance reliability.
Machine Learning and AI in Autonomy
Machine learning and AI are at the heart of self-driving cars, enabling advanced object recognition and predictive behavior modeling. These technologies play crucial roles in interpreting and navigating real-world environments.
Deep Learning for Object Recognition
Deep learning techniques empower autonomous vehicles to identify and classify objects in their surroundings. Using convolutional neural networks (CNNs), self-driving cars can process vast amounts of visual data, distinguishing between pedestrians, vehicles, traffic signals, and other road elements. This capability is essential for safe navigation and accident prevention.
These systems require extensive training using labeled datasets to improve accuracy. Companies often employ synthetic data to augment real-world data, enhancing the network’s ability to recognize objects under varying conditions. Improving object recognition is key to progressing autonomy levels in vehicles.
Data-Driven Behavior Prediction
Predictive modeling is crucial for anticipating the movements and actions of other road users. Machine learning algorithms analyze historical data to predict behaviors such as lane changes, stops, and turns. These predictions allow the autonomous system to make proactive decisions, avoiding potential collisions and improving traffic flow.
Data is continuously gathered from vehicle sensors and external sources, enhancing model accuracy over time. Real-time data processing ensures that the vehicle adapts to dynamic driving conditions, maintaining safety and efficiency. Accurate behavior prediction underpins the reliability of self-driving technologies.